Lifting the data treasure
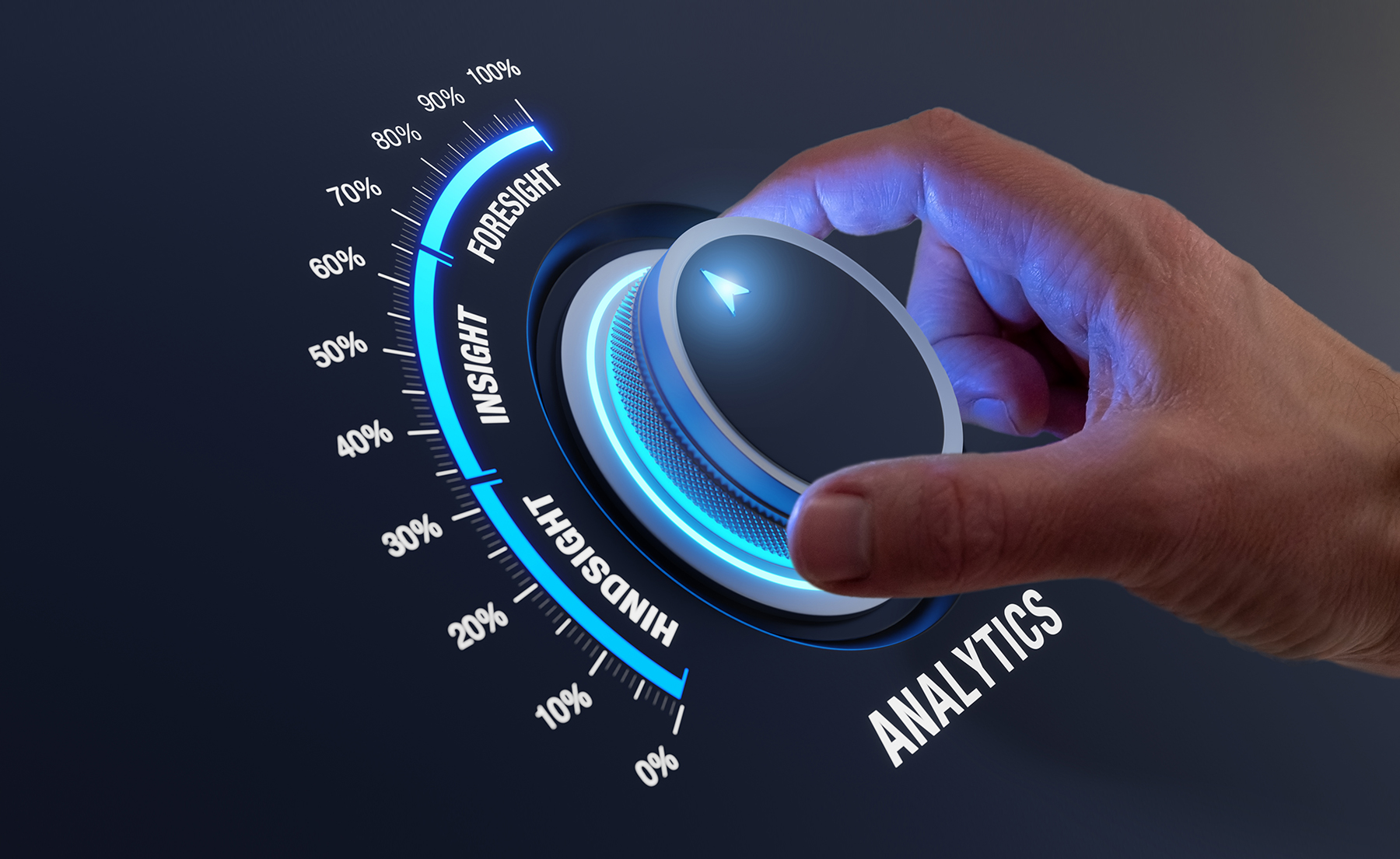
“If you know in good time when equipment is going to fail, productivity losses can be avoided through predictive maintenance,” says Bernhard Falkner (CTO Industrie Informatik) about one of the practical use cases of predictive analytics for uncovering potential savings on the shop floor. Industrie Informatik offers MES users the possibility to take proactive measures to avoid costly machine failures instead of reacting after the fact.
Using innovative approaches from the field of machine learning, it is possible, for example, to forecast the probability of a workplace malfunction in the next shift – with or without maintenance consideration – in the environment of highly networked and closely timed production processes.
Predictive analytics on the shop floor
Digital information from the manufacturing world, in combination with suitable AI methods, makes it possible to recognise patterns in it and draw appropriate conclusions about expected conditions and future trends. “The early detection of deviations based on reliable forecasts, for example on relative rejects and the quality status after production steps, is an important step towards improved capacity utilisation, adherence to schedules and lower inventory levels. Predictive analytics thus makes a significant contribution to a sustainable, digital manufacturing world,” says Falkner, describing further predictive application possibilities for medium-sized companies on the shop floor.
From machine data to prediction
There is no question that artificial intelligence in manufacturing offers numerous added values. However, users initially face hurdles with regard to the complexity resulting from the need to provide and process data. According to the German study “Künstliche Intelligenz – Wo steht die deutsche Wirtschaft – Artificial Intelligence – Where does the German economy stand?” commissioned by the digital association Bitkom (source: Bitkom Research 2022), “the biggest obstacles to the use of AI in companies are currently a lack of human resources and a lack of data for AI use (62 per cent each)”.
In order to be able to use these new technologies quickly and profitably, Industrie Informatik has therefore chosen the path of an out-of-the-box technology stack that enables an easy entry into the topics of artificial intelligence and predictive analytics. “While AI projects often require up to 80 per cent of the time for data preparation, we have already implemented the majority of this for our MES users and included it in the reference architecture based on Docker technology. In addition, the use of the Random Forest Model as a learning algorithm delivers fast processing times and very good interpretability of the results,” says CTO Bernhard Falkner, dispelling many reservations about AI projects.
Specifically, a data preprocessing framework helps the user to cleanse and prepare existing plant data, machine and process data from cronetwork MES and other sources, such as time series, in the first step before an AI carries out the ongoing evaluation and interpretation of the information obtained from it. The standard data model of cronetwork MES as well as cronetwork PIDO as the central data procurement and display object create great efficiency advantages, which can be seen in the rapid implementation.
Basis for valid results
Artificial intelligence is generally said to require large amounts of data that map the behaviour of plants and processes in different operating states. A concrete quantification in terms of data sets required for the reasonable use of machine learning in manufacturing is hardly possible, as this has to be assessed in practice on a case-by-case basis. First of all, it is important to understand that the amount of data depends on various factors, such as the complexity of the problem, the structure and distribution as well as the quality of the data.
Bernhard Falkner explains:
It is more purposeful to have few, but relevant and high-quality information available than a lot of irrelevant data. Therefore, the selection of sources and data quality should always be prioritised.
At the beginning of a predictive analytics project, Industrie Informatik therefore works intensively with the user in the context of data analysis and exploration to answer the questions “Is the right data being collected in the necessary quality and are there any particularly important measured variables to be considered in this use case?”
Predictive analytics and AI play a significant role on the path to the smart factory and create unimagined opportunities to generate knowledge from the enormous amounts of data in modern production and MES systems and to minimise inefficiencies. It is therefore not surprising that 80 percent of the participants in the above-mentioned Bitkom study consider their future use in production to be probable.
Flow chart of a prediction in cronetwork MES
#1 data selection & exploration:
Pre-selection of relevant data using cronetwork PIDO.
#2 learning phase:
Based on the existing data, the model is trained and stored. The frequency of training depends on the use case, e.g. weekly or monthly.
#3 prediction phase:
The model is loaded from the storage, combined with data from the current situation and a corresponding prediction is calculated.
#4 use:
By means of web services, the prediction can be used, for example, in portals on the shop floor or the cronetwork master portal and thus serve as a basis for proactive measures in the daily production process.