AI & detailed planning
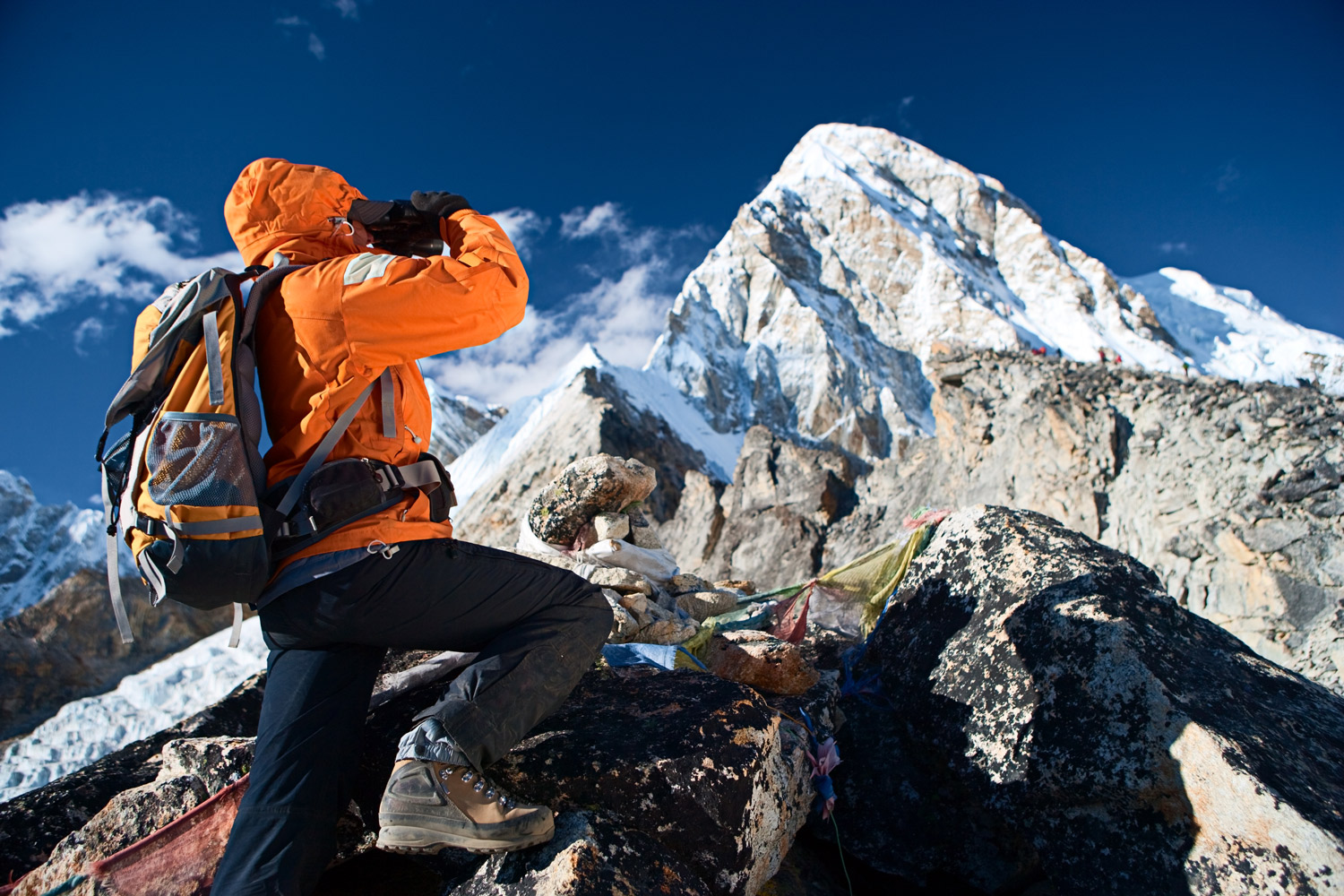
How artificial intelligence can optimize production planning
Artificial intelligence (AI) is one of the key technologies on the road to the smart factory. It is continuously finding its way into the most diverse areas of the digital manufacturing world. One of these is detailed production planning, which can benefit greatly from AI due to its inherent complexity.
However, at the outset we question how AI can be used in detailed planning and what benefits a company can derive from AI.
So let’s search for the highest peaks, or the best planning results.
Searching for the highest peak
The Alps extend over eight countries, with a total length of about 1200 km and a total area of more than 200,000 km². If we seek the highest peak, Mont Blanc, without a map and compass – i.e. practically blind – this task seems almost unsolvable. At first glance, this analogy seems far-fetched, but the connection with detailed planning will become apparent in the following.
Classic detailed production planning is characterized by a high degree of complexity. With as few as 30 operations, there are more possible arrangements than there are water molecules on earth. In addition, there are countless constraints and further dependencies on factors such as changing operation durations, varying setup times and fluctuating resource availability.
Digital scheduling boards provide a solid basis for decision-making for planners in industrial plants. So why do we need the support of an AI when existing planning tools perform well and we can also rely on the knowledge of the production planner? The answer to this question is complex and cannot be formulated in a single sentence.
We begin with learning
MES software vendor Industrie Informatik has tackled this question and searched for the next generation planning algorithms in a research project. Bernhard Falkner is CTO of the software company and is primarily responsible for the research project: “It was and is our endeavor to reproduce certain decision-making structures of humans by means of AI in order to then apply them in detailed production planning. Put simply, it is – as so often – a matter of extracting knowledge from data.”
The basis for an AI application is knowledge, or artificially generated knowledge that comes from real processes and experiences in production planning. In machine learning, planning actions and their results are calculated and then evaluated in an optimizer. These evaluations are performed by complex algorithms that use a wide variety of objective functions as their basis. Detailed planners can draw on a wide range of these objective functions, such as cost reduction, shortening of order throughput time, optimization of setup time and costs, minimization of order delays, etc.
“Industrial companies usually pursue more than one optimization objective. These must be coordinated and tracked in parallel via weighted objective functions. Only then can an AI solution meet the complex requirements of a smart factory,” says Falkner.
Hiking boots or crampons?
With an initial planning situation and the defined overall target functions as a basis, the optimizer can now get to work. This means that suboptimal and critical situations in the process are automatically detected and analyzed. Precisely here improvements are made using neighborhood operators, and a new planning screen is calculated on the basis of these improvements.
Here we return to our mountaineering example: If the goal is to climb an ice wall, a set of equipment (neighborhood operators) lends itself to this task. Depending on whether we choose hiking boots, rope, ice axe and/or crampons, we will reach our goal – or not. And the speed of ascent also varies with the equipment chosen. It all comes down to the right equipment at the right time: as dangerous and inadequate as hiking boots are on ice, their use on the alpine meadow is tried and tested.
Back to the manufacturing world: if the result improves with the neighborhood operator used, it is evaluated positively and is more likely to be used again in future simulations. The same principle applies in the other direction: If a situation does not improve as a result of the adjustments, this neighborhood operator is used less frequently.
Bernhard Falkner sees this as the possibility of near perfect detailed production planning: “The longer you let the optimizer run, the more planning scenarios it runs through and the higher the probability of an optimal planning result.”
AI in detailed planning practice
How do we use AI and, in this particular case, the optimizer in practice? The new technologies should by no means be seen as a substitute for planning personnel, but should make the work of the employees easier and significantly increase efficiency. In fact, the optimizer initiates a background process that starts its work parallel to the running production, runs through scenario by scenario on the basis of the latest scientific algorithms, and evaluates the results by means of target functions. As a user, you have can view the current status of planning and the evaluation of the target function at any time and decide when to shift to real operation.
The more time you give the optimizer, the closer you get to the “perfect” planning result. “The application possibilities are multifaceted. For example, you can also track different target functions in simulations running in parallel and thus set up further comparisons,” Bernhard Falkner says, convinced of the positive effects.
Leveraging potential benefits or finding the highest peak
An essential success factor is the global solution search of the optimizer. It does not limit itself to a local optimum, but includes solution paths that a human planner could not grasp by hand. Back in the Alps, this means that in our search for the highest peak, we may already be standing on the 3,798 m high Großglockner and believe that we have reached our goal. In truth, however, there are still many higher peaks, so there is still room for improvement.